Blog
Planning systems supported by algorithms and the often essential intermediate step
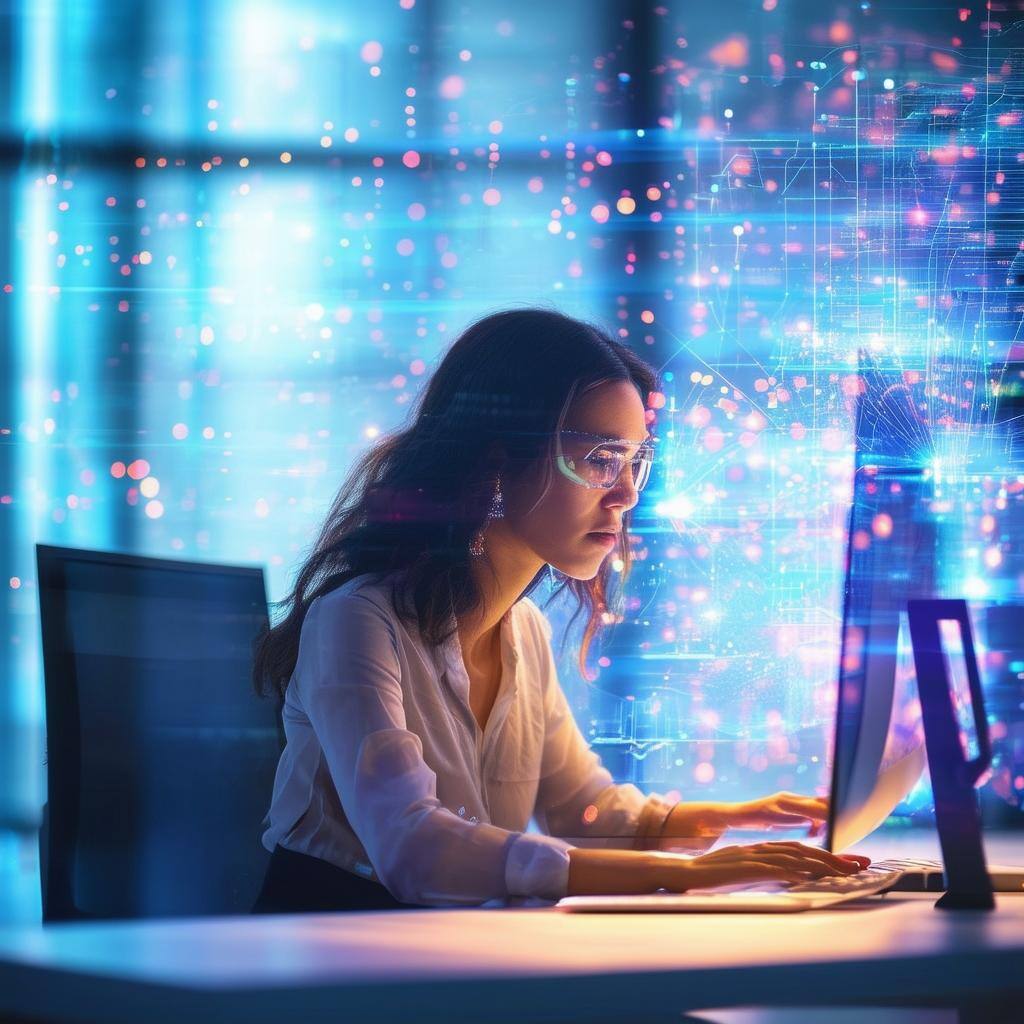
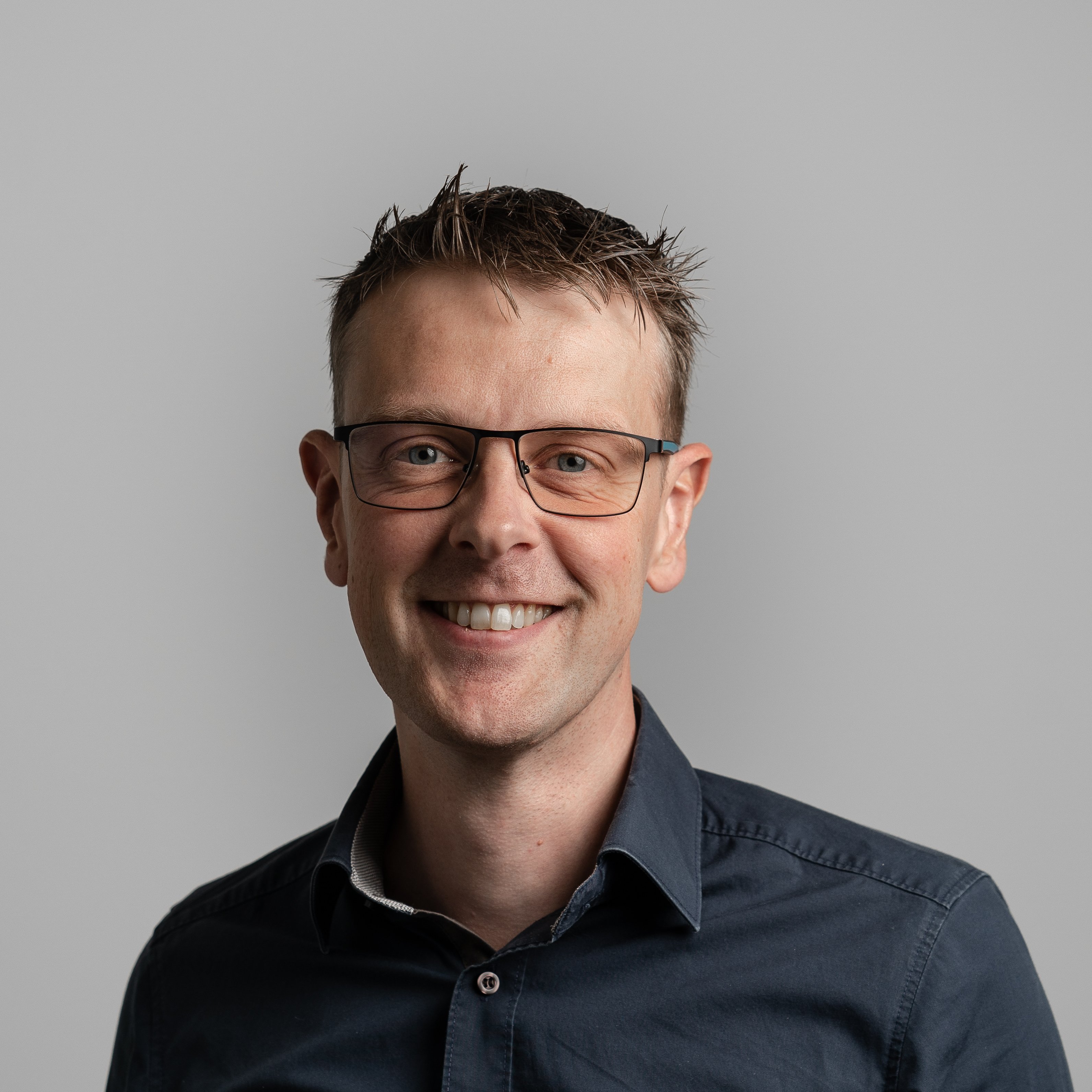
Dennis Ostendorf
Published on November 13, 2024
For decades, companies have been applying mathematical methods to automate and optimize schedules and rosters. With the rise of AI, expectations have risen even further. In doing so, entrepreneurs dream of planners who no longer have to puzzle. At the touch of a button, the computer produces the best plan. But is that so?
'Jack would rather not work on Fridays'
In practice, this planning at the push of a button often proves difficult. And this is not necessarily due to technology. Algorithms are excellent at finding optimal solutions to well-defined problems. But therein lies the challenge: the problem must be well defined. This means that all scheduling rules must be complete and clear and the input data structured, complete and correct. This is exactly where things go wrong. Rules that make sense to a human planner are difficult to capture in a strict algorithmic framework. Just think of knowledge such as "Jack prefers not to work on Fridays. Moreover, daily data is often not good enough to leave planning entirely to a computer.
Less effort for better schedules
Optimization of scheduling and rosters is ultimately not just about using AI and smart algorithms. These can be very powerful, but at the same time are not an end in themselves. Ultimately, it is about making better schedules with less effort. In other words, achieving the best possible results with the least amount of effort. As many companies and planners know by now, mathematical techniques can play an important role in this. Yet those techniques are not always directly essential to achieving significant improvements.
From intermediate step to the 'More Optimal' step
The More Optimal planning application can already add a lot of value to planning problems that are not yet ready for mathematical algorithms. The software does that, among other things, by visualizing planning problems in a clear way and making the consequences of decisions understandable. At the same time, the use of a good, decision-supporting, planning application reduces the step to the next phase: the use of optimization algorithms that further support the planner.
Practical example: Excel
As More Optimal recently implemented a scheduling application at a client. This company previously worked with several Excel sheets. They were able to plan new orders quite well. The planner usually knew who was available for which job and kept track of it in Excel. The real challenge came with changes in the planning. If a job was rescheduled for a week, it was difficult to find a suitable solution. The planner lacked an overview of who was available when, who had the right skills and how much travel time was needed. To still maintain some overview, the planner had to make changes manually in several places.
Transition to planner with insight
After the transition to the new planning system, this all became easier. The planner can now see at a glance who is doing what job at what time. The application gives an instant warning if someone does not have the right skills and calculates travel times automatically. This makes it easy for the planner to choose the best candidate for the job. This can be done both during initial planning and when disruptions occur. In time, we still want to apply mathematical optimization and thus take the next step, but first the basics have to be on order. In this case, that's making sure we have good manual scheduling in a powerful scheduling application.